208 billion transistors, Nvidia launches the most powerful AI chip GB200
Currently, NVIDIA sits at the pinnacle of the artificial intelligence world, possessing data center GPUs that everyone desires. Its Hopper H100 and GH200 Grace Hopper superchips are in high demand, powering many of the world's most powerful supercomputers.
Today, CEO Jen-Hsun Huang dropped the Blackwell B200 bombshell, which is the next-generation data center and AI GPU, offering a massive generational leap in computing power.
The Blackwell architecture and B200 GPU replace the H100/H200. Blackwell consists of three parts: B100, B200, and the Grace-Blackwell Superchip (GB200).
New Generation AI Chip BLACKWELL GPU
The new B200 GPU boasts 208 billion transistors, delivering up to 20 petaflops of FP4 compute power, while the GB200 combines two GPUs with a Grace CPU to provide 30 times the performance for LLM inference workloads, potentially also significantly increasing efficiency. NVIDIA claims that compared to the H100, its cost and energy consumption can be "reduced by up to 25 times."
NVIDIA asserts that training a model with 1.8 trillion parameters previously required 8,000 Hopper GPUs and 15 megawatts of electricity. Now, 2,000 Blackwell GPUs can accomplish this task, consuming only 4 megawatts of power.
Advertisement
In the GPT-3 LLM benchmark test with 175 billion parameters, the GB200's performance is 7 times that of the H100, and NVIDIA claims its training speed is 4 times faster than the H100.
The Blackwell B200 is not a traditional single GPU in the usual sense. Instead, it consists of two closely coupled chips that, according to NVIDIA, do act as a unified CUDA GPU. These two chips are connected via a 10 TB/s NV-HBI (NVIDIA High Bandwidth Interface) to ensure they operate as a single fully coherent chip.
The reason for this dual-chip configuration is straightforward: the Blackwell B200 will utilize TSMC's 4NP process node, an improved version of the 4N process used by existing Hopper H100 and Ada Lovelace architecture GPUs.
The B200 will employ two full die-size chips, each with four HMB3e stacks, each stack having a capacity of 24GB, and each stack featuring a 1 TB/s bandwidth over a 1024-bit interface.NVIDIA NVLink 7.2T
A significant limiting factor for AI and HPC workloads is the multi-node interconnect bandwidth between different nodes. As the number of GPUs increases, communication becomes a severe bottleneck, consuming up to 60% of resources and time. With the B200, NVIDIA introduces the fifth-generation NVLink and NVLink Switch 7.2T.
The new NVLink chip features a full-duplex bidirectional bandwidth of 1.8 TB/s, supporting 576 GPU NVLink domains. It is a 50 billion transistor chip manufactured on the same TSMC 4NP node. The chip also supports 3.6 teraflops of Sharp v4 on-chip network computing, which aids in efficiently handling larger models.
The previous generation supported up to 100 GB/s of HDR InfiniBand bandwidth, so this is a massive leap in bandwidth. Compared to the H100 multi-node interconnect, the new NVSwitch is 18 times faster. This should significantly improve the scalability of larger trillion-parameter model AI networks.
Related to this, each Blackwell GPU is equipped with 18 fifth-generation NVLink connections. This is eighteen times the number of H100 links. Each link provides 50 GB/s of bidirectional bandwidth, or 100 GB/s per link.
NVIDIA B200 NVL72
Combining the above, you get NVIDIA's new GB200 NVL72 system.
These are essentially a full-rack solution with 18 1U servers, each server housing two GB200 super chips. However, there are some differences in the composition of the GB200 super chips compared to the previous generation. Images and specifications indicate that two B200 GPUs are matched with a single Grace CPU, while GH100 uses a smaller solution, pairing a single Grace CPU with a single H100 GPU.
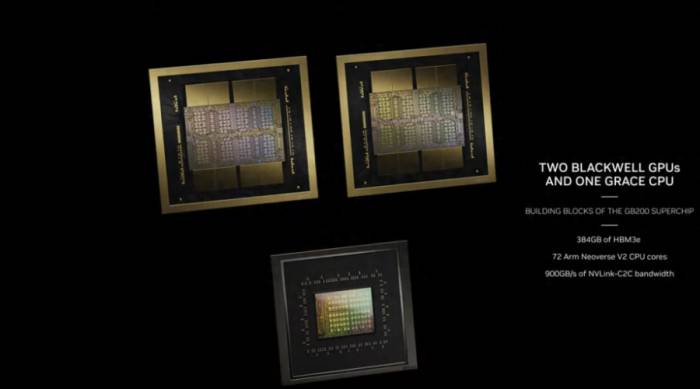
The end result is that the GB200 super chip compute tray will be equipped with two Grace CPUs and four B200 GPUs, offering 80 petaflops of FP4 AI inference performance and 40 petaflops of FP8 AI training performance. These are liquid-cooled 1U servers, which occupy a significant portion of the typical 42 unit space provided in a rack.
In addition to the GB200 super chip compute trays, the GB200 NVL72 will also be equipped with NVLink switch trays. These are also 1U liquid-cooled trays, with two NVLink switches per tray and nine such trays per rack. Each tray provides a total bandwidth of 14.4 TB/s, complemented by the aforementioned Sharp v4 computing.In summary, the GB200 NVL72 boasts 36 Grace CPUs and 72 Blackwell GPUs, with a computational capability of 720 petaflops in FP8 and 1,440 petaflops in FP4. The multi-node bandwidth is 130 TB/s, and Nvidia claims that the NVL72 can handle AI LLM models with up to 270 trillion parameters.
Nvidia has stated that Amazon, Google, Microsoft, and Oracle have all planned to offer NVL72 racks within their cloud service products.
How does the Blackwell platform perform?
While Nvidia dominates the artificial intelligence infrastructure market, it is not the only company making moves. Intel and AMD have introduced new Gaudi and Instinct accelerators, cloud providers are pushing for custom chips, and AI startups like Cerebras and Samba Nova are all vying for a share of the AI market.
It is projected that by 2024, the demand for AI accelerators will far outstrip supply. Winning market share does not always mean having the fastest chips, but simply having deliverable chips.
Although we know little about Intel's upcoming Guadi 3 chip, we can make some comparisons with AMD's MI300X GPU, which was launched in December last year.
The MI300X uses advanced packaging to vertically stack eight CDNA 3 compute units onto four I/O chips, providing high-speed communication between the GPU and 192GB of HBM3 memory.
In terms of performance, the MI300X has a 30% performance advantage over Nvidia's H100 in FP8 floating-point computations and nearly a 2.5 times lead in HPC-centric double-precision workloads.
Comparing the 750W MI300X with the 700W B100, Nvidia's chip has 2.67 times faster sparse performance. Although both chips now come with 192 GB of high-bandwidth memory, the memory speed in the Blackwell segment is 2.8 TB/s faster.
Memory bandwidth has proven to be a key indicator of AI performance, especially in inference. Nvidia's H200 is essentially an H100 with enhanced bandwidth. However, despite having the same FLOPS as the H100, Nvidia claims that it is twice as fast in models such as Meta's Llama 2 70B.Although Nvidia has a clear lead in lower precision, this may come at the expense of double precision performance, an area where AMD has excelled in recent years, winning several high-profile supercomputer awards.
According to Nvidia, the Blackwell GPU is capable of delivering 45 teraFLOPS of FP64 tensor core performance. This is a slight decrease compared to the 67 teraFLOPS of FP64 matrix performance offered by the H100, and it is at a disadvantage compared to AMD's MI300X (81.7 teraFLOPS of FP64 vector and 163 teraFLOPS of FP64 matrix).
There is also Cerebras, which recently showcased its third-generation Waferscale AI accelerator. The monster 900,000-core processor, the size of a dinner plate, is designed specifically for AI training.
Cerebras claims that each of these chips can achieve highly sparse FP16 performance of 125 petaFLOPS at a power of 23kW. Cerebras states that the chip is about 62 times faster at half-precision compared to the H100.
However, when comparing the WSE-3 to Nvidia's flagship Blackwell component, the lead narrows significantly. As far as we understand, Nvidia's top-spec chip should be able to deliver approximately 5 petaFLOPS of sparse FP16 performance. This reduces Cerebras's lead to 25 times. But as we pointed out at the time, all of this depends on whether your model can take advantage of sparsity.
TSMC and Synopsys are advancing the deployment of Nvidia's computational lithography platform.
Nvidia announced today that TSMC and Synopsys will use Nvidia's computational lithography platform for production to accelerate manufacturing and break through the physical limits of next-generation advanced semiconductor chips.
The world-leading foundry TSMC and the leader in chip-to-system design solutions, Synopsys, have integrated Nvidia's cuLitho with their software, manufacturing processes, and system integration to speed up chip manufacturing and support the latest generation of Nvidia Blackwell architecture GPUs in the future.
Nvidia founder and CEO Jen-Hsun Huang said, "Computational lithography is the cornerstone of chip manufacturing." "We are working with TSMC and Synopsys on cuLitho, applying accelerated computing and generative AI to open up new frontiers in semiconductor scaling."
Nvidia also launched a new generative AI algorithm, enhancing cuLitho (GPU-accelerated computational lithography library), which significantly improves semiconductor manufacturing processes compared to current CPU-based methods.Computational lithography is the most compute-intensive workload in semiconductor manufacturing, consuming billions of CPU hours annually. A typical mask set for chips, a key step in their production, may require 30 million or more hours of CPU computation time, necessitating the establishment of large data centers within semiconductor foundries. By accelerating computation, 350 NVIDIA H100 systems can now replace 40,000 CPU systems, speeding up production time while reducing costs, space, and power consumption.
Dr. CC Wei, CEO of TSMC, said: "By integrating GPU-accelerated computing into TSMC's workflows in collaboration with NVIDIA, we have achieved a significant leap in performance, a substantial increase in throughput, a reduction in cycle time, and a decrease in power consumption requirements." "We are transitioning NVIDIA's cuLitho to TSMC production, leveraging this computational lithography technology to drive key components of semiconductor miniaturization."
Since its launch last year, cuLitho has opened up new opportunities for TSMC in innovative patterning technology. When testing cuLitho on a shared workflow, the two companies jointly achieved a 45-fold acceleration of the curve process and nearly a 60-fold improvement in the traditional Manhattan process. These two types of flows are different; for curves, the mask shape is represented by curves, while Manhattan mask shapes are limited to horizontal or vertical.
Sassine Ghazi, President and CEO of Synopsys, said: "For over two decades, Synopsys' Proteus mask synthesis software product has been the production-proven choice for accelerating computational lithography, the most demanding workload in semiconductor manufacturing." "As the transition to advanced nodes occurs, the complexity and computational cost of computational lithography increase dramatically. Our collaboration with TSMC and NVIDIA is crucial for achieving angstrom-scale expansion, as we pioneer advanced technologies that reduce turnaround time by several orders of magnitude through the power of accelerated computing."
*Disclaimer: This article is the original creation of the author. The content of the article represents their personal views, and our reposting is solely for sharing and discussion, not an endorsement or agreement. If you have any objections, please contact the backend.
Currently, NVIDIA sits at the pinnacle of the artificial intelligence world, possessing data center GPUs that everyone desires. Its Hopper H100 and GH200 Grace Hopper superchips are in high demand, powering many of the world's most powerful supercomputers.
Today, CEO Jen-Hsun Huang dropped the Blackwell B200 bombshell, which is the next-generation data center and AI GPU, offering a massive generational leap in computing power.
The Blackwell architecture and B200 GPU replace the H100/H200. Blackwell consists of three parts: B100, B200, and the Grace-Blackwell Superchip (GB200).
New Generation AI Chip BLACKWELL GPU
The new B200 GPU boasts 208 billion transistors, delivering up to 20 petaflops of FP4 compute power, while the GB200 combines two GPUs with a Grace CPU to provide 30 times the performance for LLM inference workloads, potentially also significantly increasing efficiency. NVIDIA claims that compared to the H100, its cost and energy consumption can be "reduced by up to 25 times."
NVIDIA asserts that training a model with 1.8 trillion parameters previously required 8,000 Hopper GPUs and 15 megawatts of electricity. Now, 2,000 Blackwell GPUs can accomplish this task, consuming only 4 megawatts of power.
Advertisement
In the GPT-3 LLM benchmark test with 175 billion parameters, the GB200's performance is 7 times that of the H100, and NVIDIA claims its training speed is 4 times faster than the H100.
The Blackwell B200 is not a traditional single GPU in the usual sense. Instead, it consists of two closely coupled chips that, according to NVIDIA, do act as a unified CUDA GPU. These two chips are connected via a 10 TB/s NV-HBI (NVIDIA High Bandwidth Interface) to ensure they operate as a single fully coherent chip.
The reason for this dual-chip configuration is straightforward: the Blackwell B200 will utilize TSMC's 4NP process node, an improved version of the 4N process used by existing Hopper H100 and Ada Lovelace architecture GPUs.
The B200 will employ two full die-size chips, each with four HMB3e stacks, each stack having a capacity of 24GB, and each stack featuring a 1 TB/s bandwidth over a 1024-bit interface.NVIDIA NVLink 7.2T
A significant limiting factor for AI and HPC workloads is the multi-node interconnect bandwidth between different nodes. As the number of GPUs increases, communication becomes a severe bottleneck, consuming up to 60% of resources and time. With the B200, NVIDIA introduces the fifth-generation NVLink and NVLink Switch 7.2T.
The new NVLink chip features a full-duplex bidirectional bandwidth of 1.8 TB/s, supporting 576 GPU NVLink domains. It is a 50 billion transistor chip manufactured on the same TSMC 4NP node. The chip also supports 3.6 teraflops of Sharp v4 on-chip network computing, which aids in efficiently handling larger models.
The previous generation supported up to 100 GB/s of HDR InfiniBand bandwidth, so this is a massive leap in bandwidth. Compared to the H100 multi-node interconnect, the new NVSwitch is 18 times faster. This should significantly improve the scalability of larger trillion-parameter model AI networks.
Related to this, each Blackwell GPU is equipped with 18 fifth-generation NVLink connections. This is eighteen times the number of H100 links. Each link provides 50 GB/s of bidirectional bandwidth, or 100 GB/s per link.
NVIDIA B200 NVL72
Combining the above, you get NVIDIA's new GB200 NVL72 system.
These are essentially a full-rack solution with 18 1U servers, each server housing two GB200 super chips. However, there are some differences in the composition of the GB200 super chips compared to the previous generation. Images and specifications indicate that two B200 GPUs are matched with a single Grace CPU, while GH100 uses a smaller solution, pairing a single Grace CPU with a single H100 GPU.
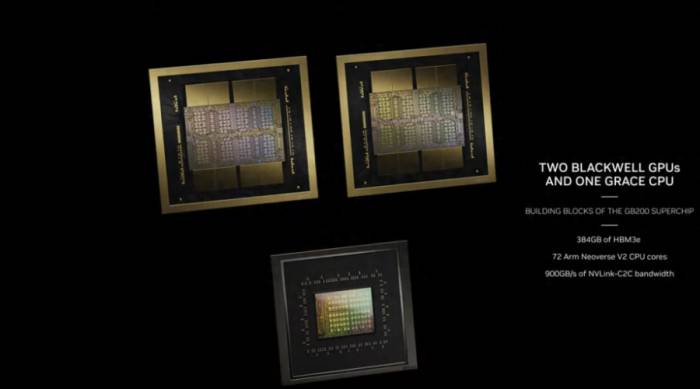
The end result is that the GB200 super chip compute tray will be equipped with two Grace CPUs and four B200 GPUs, offering 80 petaflops of FP4 AI inference performance and 40 petaflops of FP8 AI training performance. These are liquid-cooled 1U servers, which occupy a significant portion of the typical 42 unit space provided in a rack.
In addition to the GB200 super chip compute trays, the GB200 NVL72 will also be equipped with NVLink switch trays. These are also 1U liquid-cooled trays, with two NVLink switches per tray and nine such trays per rack. Each tray provides a total bandwidth of 14.4 TB/s, complemented by the aforementioned Sharp v4 computing.In summary, the GB200 NVL72 boasts 36 Grace CPUs and 72 Blackwell GPUs, with a computational capability of 720 petaflops in FP8 and 1,440 petaflops in FP4. The multi-node bandwidth is 130 TB/s, and Nvidia claims that the NVL72 can handle AI LLM models with up to 270 trillion parameters.
Nvidia has stated that Amazon, Google, Microsoft, and Oracle have all planned to offer NVL72 racks within their cloud service products.
How does the Blackwell platform perform?
While Nvidia dominates the artificial intelligence infrastructure market, it is not the only company making moves. Intel and AMD have introduced new Gaudi and Instinct accelerators, cloud providers are pushing for custom chips, and AI startups like Cerebras and Samba Nova are all vying for a share of the AI market.
It is projected that by 2024, the demand for AI accelerators will far outstrip supply. Winning market share does not always mean having the fastest chips, but simply having deliverable chips.
Although we know little about Intel's upcoming Guadi 3 chip, we can make some comparisons with AMD's MI300X GPU, which was launched in December last year.
The MI300X uses advanced packaging to vertically stack eight CDNA 3 compute units onto four I/O chips, providing high-speed communication between the GPU and 192GB of HBM3 memory.
In terms of performance, the MI300X has a 30% performance advantage over Nvidia's H100 in FP8 floating-point computations and nearly a 2.5 times lead in HPC-centric double-precision workloads.
Comparing the 750W MI300X with the 700W B100, Nvidia's chip has 2.67 times faster sparse performance. Although both chips now come with 192 GB of high-bandwidth memory, the memory speed in the Blackwell segment is 2.8 TB/s faster.
Memory bandwidth has proven to be a key indicator of AI performance, especially in inference. Nvidia's H200 is essentially an H100 with enhanced bandwidth. However, despite having the same FLOPS as the H100, Nvidia claims that it is twice as fast in models such as Meta's Llama 2 70B.Although Nvidia has a clear lead in lower precision, this may come at the expense of double precision performance, an area where AMD has excelled in recent years, winning several high-profile supercomputer awards.
According to Nvidia, the Blackwell GPU is capable of delivering 45 teraFLOPS of FP64 tensor core performance. This is a slight decrease compared to the 67 teraFLOPS of FP64 matrix performance offered by the H100, and it is at a disadvantage compared to AMD's MI300X (81.7 teraFLOPS of FP64 vector and 163 teraFLOPS of FP64 matrix).
There is also Cerebras, which recently showcased its third-generation Waferscale AI accelerator. The monster 900,000-core processor, the size of a dinner plate, is designed specifically for AI training.
Cerebras claims that each of these chips can achieve highly sparse FP16 performance of 125 petaFLOPS at a power of 23kW. Cerebras states that the chip is about 62 times faster at half-precision compared to the H100.
However, when comparing the WSE-3 to Nvidia's flagship Blackwell component, the lead narrows significantly. As far as we understand, Nvidia's top-spec chip should be able to deliver approximately 5 petaFLOPS of sparse FP16 performance. This reduces Cerebras's lead to 25 times. But as we pointed out at the time, all of this depends on whether your model can take advantage of sparsity.
TSMC and Synopsys are advancing the deployment of Nvidia's computational lithography platform.
Nvidia announced today that TSMC and Synopsys will use Nvidia's computational lithography platform for production to accelerate manufacturing and break through the physical limits of next-generation advanced semiconductor chips.
The world-leading foundry TSMC and the leader in chip-to-system design solutions, Synopsys, have integrated Nvidia's cuLitho with their software, manufacturing processes, and system integration to speed up chip manufacturing and support the latest generation of Nvidia Blackwell architecture GPUs in the future.
Nvidia founder and CEO Jen-Hsun Huang said, "Computational lithography is the cornerstone of chip manufacturing." "We are working with TSMC and Synopsys on cuLitho, applying accelerated computing and generative AI to open up new frontiers in semiconductor scaling."
Nvidia also launched a new generative AI algorithm, enhancing cuLitho (GPU-accelerated computational lithography library), which significantly improves semiconductor manufacturing processes compared to current CPU-based methods.Computational lithography is the most compute-intensive workload in semiconductor manufacturing, consuming billions of CPU hours annually. A typical mask set for chips, a key step in their production, may require 30 million or more hours of CPU computation time, necessitating the establishment of large data centers within semiconductor foundries. By accelerating computation, 350 NVIDIA H100 systems can now replace 40,000 CPU systems, speeding up production time while reducing costs, space, and power consumption.
Dr. CC Wei, CEO of TSMC, said: "By integrating GPU-accelerated computing into TSMC's workflows in collaboration with NVIDIA, we have achieved a significant leap in performance, a substantial increase in throughput, a reduction in cycle time, and a decrease in power consumption requirements." "We are transitioning NVIDIA's cuLitho to TSMC production, leveraging this computational lithography technology to drive key components of semiconductor miniaturization."
Since its launch last year, cuLitho has opened up new opportunities for TSMC in innovative patterning technology. When testing cuLitho on a shared workflow, the two companies jointly achieved a 45-fold acceleration of the curve process and nearly a 60-fold improvement in the traditional Manhattan process. These two types of flows are different; for curves, the mask shape is represented by curves, while Manhattan mask shapes are limited to horizontal or vertical.
Sassine Ghazi, President and CEO of Synopsys, said: "For over two decades, Synopsys' Proteus mask synthesis software product has been the production-proven choice for accelerating computational lithography, the most demanding workload in semiconductor manufacturing." "As the transition to advanced nodes occurs, the complexity and computational cost of computational lithography increase dramatically. Our collaboration with TSMC and NVIDIA is crucial for achieving angstrom-scale expansion, as we pioneer advanced technologies that reduce turnaround time by several orders of magnitude through the power of accelerated computing."
*Disclaimer: This article is the original creation of the author. The content of the article represents their personal views, and our reposting is solely for sharing and discussion, not an endorsement or agreement. If you have any objections, please contact the backend.