Autonomous driving technology has suffered a Waterloo
In recent years, represented by Tesla CEO Musk, executives from several global automotive manufacturers have touted their Advanced Driver-Assistance Systems (ADAS) with almost mythical capabilities. However, the reality is filled with recurring accidents and potential hazards, along with multiple large-scale vehicle recalls. This has made it increasingly clear that ADAS technology is still immature and has many issues that need to be addressed.
On March 12th, the Insurance Institute for Highway Safety (IIHS) released its latest research showing that Tesla's Autopilot and Full Self-Driving (FSD) technologies, as well as the ADAS sold by several other major car manufacturers, received a "poor" rating. Currently, the National Highway Traffic Safety Administration (NHTSA) does not have formal standards to regulate advanced driver assistance systems, and the IIHS rated 14 ADAS from 9 car manufacturers based on its own criteria.
Among the systems tested by IIHS, only one received an "acceptable" rating. The IIHS pointed out that the ADAS from Tesla, Mercedes-Benz, BMW, Nissan, Ford, General Motors, Hyundai, and Volvo, which is owned by Geely, were generally rated as "poor." They only scored "good" on certain aspects of the IIHS tests, but their overall scores were low.
It is reported that U.S. federal regulators are investigating nearly 1,000 accidents involving the use of Tesla's ADAS. Tesla attributes the crashes to drivers who did not heed the manufacturer's warnings to pay attention to road conditions while using the Autopilot or FSD technology.
Advertisement
Recently, NHTSA stated that due to incorrect font size of warning lights, which increases the risk of accidents, Tesla recalled 2.2 million electric vehicles, a number that covers almost all vehicles in the United States, including Model S, Model X, Model 3 from 2017 to 2023, Model Y, and the 2024 Cybertruck. Three months ago, Tesla recalled 2.03 million electric vehicles in the U.S.
01
ADAS has many issues that need to be resolved
ADAS is a very complex system of hardware and software. Achieving safe assisted driving is a challenging task and not as impressive as car manufacturers currently advertise. To achieve true Level 3, or even Level 4, assisted driving, many issues need to be addressed, such as the computational power of the core processors and their software support capabilities, the enhancement of performance and reliability of other components related to ADAS, the maturation of perception technology, and the improvement of regulations and the unification of technical standards. The core processors are still in the iteration process.As the levels of assisted driving technology advance, the application functions become increasingly rich, and the demand for chip computing power in vehicles rises accordingly. Particularly in terms of safety and real-time performance, Advanced Driver-Assistance Systems (ADAS) have high requirements, necessitating systems with enhanced cognitive and reasoning capabilities.
Currently, manufacturers such as Mobileye, NVIDIA, and Tesla are at the forefront of the market in ADAS computing chips, with their products widely applied in mid-to-high-end and new energy vehicle models.
Mobileye is the leader in L2 and below-level assisted driving and is also the founder and leader in automotive ADAS technology. Before the rise of NVIDIA and Tesla, Mobileye had always been the industry leader in ADAS.
Starting with vision-based solutions, Mobileye has now also developed integrated solutions with LiDAR. Mobileye provides automakers with an assisted driving solution that includes chips and perception algorithms, with the main chip being the EyeQ. The perception algorithms are pre-installed within the EyeQ, which can directly output perception results for lane lines and vehicles. Automakers' algorithms then make driving decisions based on these results. The advantage of this approach is that it accelerates the mass production speed of automakers seeking to transform into smart vehicles. However, this method results in a slower upgrade and iteration speed of computing power, making it difficult to meet the customized needs of automakers, ultimately leading to insufficient product differentiation capabilities and an inability to meet the rapidly developing assisted driving market demands.
Due to these obvious shortcomings, starting from 2020, the shipment growth rate of EyeQ chips has significantly declined, especially in the electric vehicle sector where the level of intelligence is high. Moreover, Mobileye's closed ecosystem model struggles to meet development requirements. In the past two years, Mobileye has also recognized the issues and claimed that EyeQ5 will open up some algorithms to users, but the extent of this openness has not been clearly defined.
Currently, many traditional automakers' models still use Mobileye's solutions. When the hardware and software do not meet the requirements of advanced assisted driving, coupled with the driver's carelessness, accidents are inevitable.
NVIDIA has integrated special-function GPUs and auxiliary chips, launching the first generation of in-vehicle chips known as the Drive series. As the demands of in-vehicle systems evolve, the Drive system is also continuously upgrading. For example, the Drive PX Xavier is equipped with a Xavier chip suitable for L2-level assisted driving. For higher-level applications, the computing power of a single Xavier chip is not enough, and two Xavier chips plus two Turing architecture GPUs can be used. Additionally, NVIDIA has introduced a standalone Orin chip. Last year, the company launched Thor, which boasts a computing power of 2000 TOPS, with a single chip's computing power being eight times that of Orin. This allows customers to choose the appropriate chip for different usage scenarios.
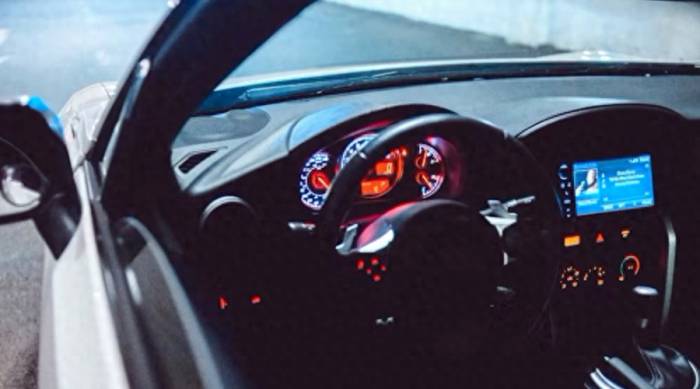
Beyond computing power, NVIDIA also places great emphasis on the development of software tools, successively launching DRIVE OS, DRIVEWORKS, DRIVE AV, and DRIVE IX.
Although NVIDIA's solutions are more intelligent and flexible compared to traditional hardware and software systems, they still cannot guarantee absolute reliability on complex road surfaces. Precisely for this reason, the company has been focusing on enhancing the computing power of core processors while continuously improving software functionality.
Recognizing the weaknesses in hardware and software products of third-party core processor suppliers represented by Mobileye and NVIDIA, Tesla has chosen to develop its own ADAS core processors and software algorithms.Tesla's ADAS processor, known as the Full Self-Driving (FSD) chip, was developed to replace the previously utilized EyeQ3 and Drive platforms. The FSD chip's design is algorithm-driven, featuring a novel chip architecture centered around two Neural Processing Units (NPUs) that form the Neural Network Accelerator (NNA). This approach, starting with the algorithm to design the chip architecture, results in a more energy-efficient performance. It also allows for more aggressive experimentation with new solutions without the need for complex processes such as third-party automotive-grade certifications. Additionally, both the hardware and software are developed in-house, which accelerates the vehicle development cycle and offers higher efficiency compared to the purchase of off-the-shelf chips.
From an architectural and performance perspective, Tesla's self-developed ADAS system exhibits strong innovation and is well-suited for contemporary assistive driving systems. However, the company's strategy is rather aggressive, implementing their ideas and systems into vehicles without sufficient testing data, which has sparked considerable controversy and a series of traffic accidents.
Overall, for autonomous driving systems, both software and hardware play extremely important roles, and neither can be omitted.
With the widespread application of AI technology, autonomous driving has entered the era of AI chips. Computational power is no longer the sole metric for measuring the level of autonomous driving; it is also essential to consider whether there are algorithms specific to a certain domain, known as domain-specific algorithms. This provides later entrants in the chip market with more opportunities to catch up with leading companies in specific areas. Even if they cannot compete in raw computational power, they can optimize domain-specific algorithms to achieve better overall performance.
For automotive manufacturers, since the level of assistive driving has not yet reached Level 3, they will not blindly pursue high computational power chips or platforms. Instead, they must consider a comprehensive set of indicators for assistive driving chips, such as computational power and efficiency, adaptability, software development difficulty, automotive-grade safety certification level, flexibility, and energy efficiency ratio, to choose the most cost-effective chips based on the vehicle's price range.
Especially in terms of safety and real-time performance, the requirements for assistive driving are very high, necessitating systems with enhanced cognitive and reasoning capabilities. At this point, the importance of software and algorithms becomes increasingly prominent and represents the core competitiveness of automotive companies, such as vision algorithms for sensor data processing and fusion, radar algorithms, and path planning, behavior decision-making, etc.
The performance and reliability of various components need to be improved.
High-level assistive driving systems require an upgrade in the performance of corresponding components, such as cameras, lidar, vehicle control chips, electronic braking systems, and Driver Monitoring Systems (DMS).
The prerequisite for the application of high-level assistive driving is the enhancement of the vehicle's perception capabilities, which will increase the demand for the installation and performance of perception equipment such as cameras, millimeter-wave radars, and lidar. Among them, cameras will evolve from low to high pixel counts, and their installation numbers will also increase driven by the demand for 360-degree surround imaging. Millimeter-wave radars and lidar can provide advanced assistive driving systems with stronger road information collection capabilities when pure vision solutions are not yet mature.
High-level assistive driving systems are more intelligent, requiring underlying chips with higher computational power, while also demanding improvements in power consumption and compatibility. In addition, the electronic and electrical (E/E) architecture of high-level assistive driving systems will tend to be centralized, with intelligent driving domain controllers and central computing centers based on a single System on Chip (SoC) gradually replacing traditional distributed ECUs. This shift places higher performance and reliability demands on the products of domain controllers and central computing centers.Compared to traditional mechanical hydraulic braking and steering, wire-controlled braking and steering offer advantages such as faster response times, high compatibility with electrified architectures, energy recovery, and the ability to configure multiple redundancy mechanisms, making them more suitable for high-level assisted driving vehicles. Currently, wire-controlled braking and steering technologies and products have not fully met safety application requirements.
Driver Monitoring Systems (DMS) are primarily used for driver identification, fatigue driving detection, and hazardous behavior monitoring. For L3-level autonomous vehicles, drivers are still required to take over the control of the vehicle in special circumstances, and some national regulations have made specific provisions regarding whether drivers can make phone calls or watch entertainment systems under L3 assisted driving conditions. These requirements necessitate the vehicle to be equipped with DMS to determine responsibility in the event of an accident. Current DMS solutions struggle to meet the demands of L3-level assisted driving.
The industry chain needs adjustment.
As higher-level assisted driving technologies mature, they will have a significant impact on the related chip component industry chain, and may even lead to a reorganization of the industry chain.
Under the traditional distributed E/E architecture, the vehicle's assisted driving system is composed of several independent subsystems (forward ADAS, side and rear ADAS, parking assistance system, panoramic surround view system), each with an ECU, and the main physical structure of the ECU is a microcontroller plus peripheral circuits. In this architecture, hardware and software are tightly coupled, with Tier1 suppliers providing "black box" delivery of integrated hardware and software to the vehicle manufacturers. As the vehicle E/E architecture transitions from distributed to centralized, the ECUs corresponding to the assisted driving subsystems also merge into an assisted driving domain controller, the main control chip evolves from an MCU to a higher-performance SoC heterogeneous chip, and the software architecture upgrades to SOA, which includes three parts: system software (virtual machine, system kernel, middleware), algorithm modules, and the application layer, achieving hardware-software decoupling. Consequently, the entire assisted driving system industry chain is also broken down into several major segments: chips, hardware integration and production, software development, algorithm development, and applications.
In the early stages of industry transformation, segments such as chips, middleware, and algorithm development have each spawned a number of startups. At this time, the core barrier for related companies lies in whether they have sufficient development capabilities and mass production experience in their respective segments. For example, in recent years, Desay SV Automotive has secured numerous orders from car manufacturers based on its mass production experience with an assisted driving domain controller based on the NVIDIA Orin chip. However, as some mid-low computing power platform domain controllers gradually become standardized, the requirements for excellent Tier1 enterprises (including chip suppliers, integration suppliers, algorithm suppliers, etc.) are no longer limited to a single segment of the industry chain. More importantly, they need to leverage their current leading advantages to fully integrate the upstream and downstream of the industry chain, and as much as possible, possess an integrated supply capability that includes chips, algorithms, manufacturing, and more.
Perception algorithms and software technology still need to advance.
Led by Tesla, vehicle manufacturers, especially those in China, have been reconstructing their perception architecture since 2022 and have proposed "heavy perception, light mapping" technical solutions. However, even with the support of a new perception architecture, the intelligent driving system still cannot rely solely on ordinary navigation maps like humans do. Currently, it only reduces the dependence on high-precision maps, rather than completely abandoning the use of any form of maps. The incremental information contained in high-precision maps can be divided into two categories: one is high-precision road geometric data (such as road width, route curvature radius, etc.), and some intelligent driving manufacturers can already supplement this information through BEV real-time perception; the other is road topology structure (such as the connection relationships between lanes, the binding of intersection traffic lights and corresponding lanes, etc.), and intelligent driving manufacturers are temporarily unable to supplement the above information solely through the BEV network architecture.
Neural network-based assisted driving models are set up with algorithm frameworks by engineers and rely on a large amount of data for parameter updates and tuning. Therefore, the level of a vehicle manufacturer's intelligent driving = algorithm construction capability * data training efficiency, where the algorithm construction capability is determined by factors such as the choice of the model itself and the technical route, and data training efficiency is determined by the vehicle manufacturer's data closed-loop capability. Currently, there are still significant differences in the maturity and iteration efficiency of data closed-loop construction among various vehicle manufacturers, so the data closed-loop capability will directly determine the vehicle manufacturer's level of intelligent driving.
The efficiency of a vehicle manufacturer's data closed-loop will be determined by its engineering capabilities. The entire data closed-loop system includes data acquisition, data preprocessing, annotation, simulation, model deployment, etc., and there are many engineering issues that need to be optimized in each link.Refine Regulations and Unify Standards
The current state of autonomous driving technology is at Level 2 (L2) and is progressing towards Level 3 (L3). Unlike L2, L3 is no longer considered an assistive driving feature but a conditional autonomous driving capability. Drivers are not required to constantly monitor the surrounding road conditions and be prepared to take over at any moment. In this case, the responsibility for driving the vehicle shifts more to the vehicle itself. Therefore, traffic accidents that occur when the autonomous driving system is functioning normally should be the responsibility of the vehicle manufacturer. However, looking at the current traffic policies of various countries, L3 autonomous driving technology has not been widely recognized, and the primary responsibility for traffic accidents is mostly clearly identified as the driver. It is evident that governments around the world are cautious about the implementation of autonomous driving, and the pace of enacting relevant laws and regulations is relatively slow, which to some extent restricts the development of higher-level autonomous driving technology.
The implementation of higher-level autonomous driving also requires countries to formulate relevant policies to provide clear answers regarding the identification standards, technical specifications, professional terminology, and evaluation systems for autonomous driving, and to establish a standard system. The establishment of a standard system is very complicated and requires continuous optimization, which poses a significant challenge for governments worldwide.
02
Conclusion
ADAS technology has been developed for many years, and there are increasingly more vehicles on the road equipped with this feature. However, safety issues have not been resolved. Moreover, some vehicles and ADAS systems are, to some extent, "experimenting" on the highway, which carries a considerable risk.
As the level of assistive driving increases, the importance of safety issues becomes more prominent. To elevate the ADAS system to a higher level of application, various hardware and software issues, intelligent perception issues, as well as the formulation of regulations and industry standards, must be thoroughly resolved.
Of course, to achieve Level 4 (L4) or even Level 5 (L5) driving functions in future consumer vehicles, in addition to technological breakthroughs, cost is an inescapable challenge. This is because to achieve L5-level driving, a wide variety of expensive hardware must be equipped on the vehicle, and the software services will not be cheap either. Whether such costs can be popularized in the lives of the general consumer is a question.
In recent years, represented by Tesla CEO Musk, executives from several global automotive manufacturers have touted their Advanced Driver-Assistance Systems (ADAS) with almost mythical capabilities. However, the reality is filled with recurring accidents and potential hazards, along with multiple large-scale vehicle recalls. This has made it increasingly clear that ADAS technology is still immature and has many issues that need to be addressed.
On March 12th, the Insurance Institute for Highway Safety (IIHS) released its latest research showing that Tesla's Autopilot and Full Self-Driving (FSD) technologies, as well as the ADAS sold by several other major car manufacturers, received a "poor" rating. Currently, the National Highway Traffic Safety Administration (NHTSA) does not have formal standards to regulate advanced driver assistance systems, and the IIHS rated 14 ADAS from 9 car manufacturers based on its own criteria.
Among the systems tested by IIHS, only one received an "acceptable" rating. The IIHS pointed out that the ADAS from Tesla, Mercedes-Benz, BMW, Nissan, Ford, General Motors, Hyundai, and Volvo, which is owned by Geely, were generally rated as "poor." They only scored "good" on certain aspects of the IIHS tests, but their overall scores were low.
It is reported that U.S. federal regulators are investigating nearly 1,000 accidents involving the use of Tesla's ADAS. Tesla attributes the crashes to drivers who did not heed the manufacturer's warnings to pay attention to road conditions while using the Autopilot or FSD technology.
Advertisement
Recently, NHTSA stated that due to incorrect font size of warning lights, which increases the risk of accidents, Tesla recalled 2.2 million electric vehicles, a number that covers almost all vehicles in the United States, including Model S, Model X, Model 3 from 2017 to 2023, Model Y, and the 2024 Cybertruck. Three months ago, Tesla recalled 2.03 million electric vehicles in the U.S.
01
ADAS has many issues that need to be resolved
ADAS is a very complex system of hardware and software. Achieving safe assisted driving is a challenging task and not as impressive as car manufacturers currently advertise. To achieve true Level 3, or even Level 4, assisted driving, many issues need to be addressed, such as the computational power of the core processors and their software support capabilities, the enhancement of performance and reliability of other components related to ADAS, the maturation of perception technology, and the improvement of regulations and the unification of technical standards. The core processors are still in the iteration process.As the levels of assisted driving technology advance, the application functions become increasingly rich, and the demand for chip computing power in vehicles rises accordingly. Particularly in terms of safety and real-time performance, Advanced Driver-Assistance Systems (ADAS) have high requirements, necessitating systems with enhanced cognitive and reasoning capabilities.
Currently, manufacturers such as Mobileye, NVIDIA, and Tesla are at the forefront of the market in ADAS computing chips, with their products widely applied in mid-to-high-end and new energy vehicle models.
Mobileye is the leader in L2 and below-level assisted driving and is also the founder and leader in automotive ADAS technology. Before the rise of NVIDIA and Tesla, Mobileye had always been the industry leader in ADAS.
Starting with vision-based solutions, Mobileye has now also developed integrated solutions with LiDAR. Mobileye provides automakers with an assisted driving solution that includes chips and perception algorithms, with the main chip being the EyeQ. The perception algorithms are pre-installed within the EyeQ, which can directly output perception results for lane lines and vehicles. Automakers' algorithms then make driving decisions based on these results. The advantage of this approach is that it accelerates the mass production speed of automakers seeking to transform into smart vehicles. However, this method results in a slower upgrade and iteration speed of computing power, making it difficult to meet the customized needs of automakers, ultimately leading to insufficient product differentiation capabilities and an inability to meet the rapidly developing assisted driving market demands.
Due to these obvious shortcomings, starting from 2020, the shipment growth rate of EyeQ chips has significantly declined, especially in the electric vehicle sector where the level of intelligence is high. Moreover, Mobileye's closed ecosystem model struggles to meet development requirements. In the past two years, Mobileye has also recognized the issues and claimed that EyeQ5 will open up some algorithms to users, but the extent of this openness has not been clearly defined.
Currently, many traditional automakers' models still use Mobileye's solutions. When the hardware and software do not meet the requirements of advanced assisted driving, coupled with the driver's carelessness, accidents are inevitable.
NVIDIA has integrated special-function GPUs and auxiliary chips, launching the first generation of in-vehicle chips known as the Drive series. As the demands of in-vehicle systems evolve, the Drive system is also continuously upgrading. For example, the Drive PX Xavier is equipped with a Xavier chip suitable for L2-level assisted driving. For higher-level applications, the computing power of a single Xavier chip is not enough, and two Xavier chips plus two Turing architecture GPUs can be used. Additionally, NVIDIA has introduced a standalone Orin chip. Last year, the company launched Thor, which boasts a computing power of 2000 TOPS, with a single chip's computing power being eight times that of Orin. This allows customers to choose the appropriate chip for different usage scenarios.
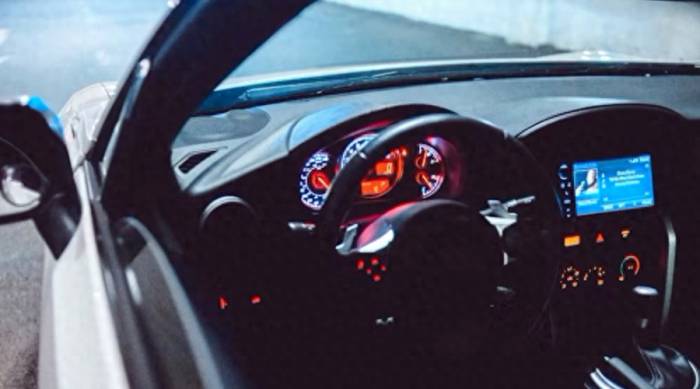
Beyond computing power, NVIDIA also places great emphasis on the development of software tools, successively launching DRIVE OS, DRIVEWORKS, DRIVE AV, and DRIVE IX.
Although NVIDIA's solutions are more intelligent and flexible compared to traditional hardware and software systems, they still cannot guarantee absolute reliability on complex road surfaces. Precisely for this reason, the company has been focusing on enhancing the computing power of core processors while continuously improving software functionality.
Recognizing the weaknesses in hardware and software products of third-party core processor suppliers represented by Mobileye and NVIDIA, Tesla has chosen to develop its own ADAS core processors and software algorithms.Tesla's ADAS processor, known as the Full Self-Driving (FSD) chip, was developed to replace the previously utilized EyeQ3 and Drive platforms. The FSD chip's design is algorithm-driven, featuring a novel chip architecture centered around two Neural Processing Units (NPUs) that form the Neural Network Accelerator (NNA). This approach, starting with the algorithm to design the chip architecture, results in a more energy-efficient performance. It also allows for more aggressive experimentation with new solutions without the need for complex processes such as third-party automotive-grade certifications. Additionally, both the hardware and software are developed in-house, which accelerates the vehicle development cycle and offers higher efficiency compared to the purchase of off-the-shelf chips.
From an architectural and performance perspective, Tesla's self-developed ADAS system exhibits strong innovation and is well-suited for contemporary assistive driving systems. However, the company's strategy is rather aggressive, implementing their ideas and systems into vehicles without sufficient testing data, which has sparked considerable controversy and a series of traffic accidents.
Overall, for autonomous driving systems, both software and hardware play extremely important roles, and neither can be omitted.
With the widespread application of AI technology, autonomous driving has entered the era of AI chips. Computational power is no longer the sole metric for measuring the level of autonomous driving; it is also essential to consider whether there are algorithms specific to a certain domain, known as domain-specific algorithms. This provides later entrants in the chip market with more opportunities to catch up with leading companies in specific areas. Even if they cannot compete in raw computational power, they can optimize domain-specific algorithms to achieve better overall performance.
For automotive manufacturers, since the level of assistive driving has not yet reached Level 3, they will not blindly pursue high computational power chips or platforms. Instead, they must consider a comprehensive set of indicators for assistive driving chips, such as computational power and efficiency, adaptability, software development difficulty, automotive-grade safety certification level, flexibility, and energy efficiency ratio, to choose the most cost-effective chips based on the vehicle's price range.
Especially in terms of safety and real-time performance, the requirements for assistive driving are very high, necessitating systems with enhanced cognitive and reasoning capabilities. At this point, the importance of software and algorithms becomes increasingly prominent and represents the core competitiveness of automotive companies, such as vision algorithms for sensor data processing and fusion, radar algorithms, and path planning, behavior decision-making, etc.
The performance and reliability of various components need to be improved.
High-level assistive driving systems require an upgrade in the performance of corresponding components, such as cameras, lidar, vehicle control chips, electronic braking systems, and Driver Monitoring Systems (DMS).
The prerequisite for the application of high-level assistive driving is the enhancement of the vehicle's perception capabilities, which will increase the demand for the installation and performance of perception equipment such as cameras, millimeter-wave radars, and lidar. Among them, cameras will evolve from low to high pixel counts, and their installation numbers will also increase driven by the demand for 360-degree surround imaging. Millimeter-wave radars and lidar can provide advanced assistive driving systems with stronger road information collection capabilities when pure vision solutions are not yet mature.
High-level assistive driving systems are more intelligent, requiring underlying chips with higher computational power, while also demanding improvements in power consumption and compatibility. In addition, the electronic and electrical (E/E) architecture of high-level assistive driving systems will tend to be centralized, with intelligent driving domain controllers and central computing centers based on a single System on Chip (SoC) gradually replacing traditional distributed ECUs. This shift places higher performance and reliability demands on the products of domain controllers and central computing centers.Compared to traditional mechanical hydraulic braking and steering, wire-controlled braking and steering offer advantages such as faster response times, high compatibility with electrified architectures, energy recovery, and the ability to configure multiple redundancy mechanisms, making them more suitable for high-level assisted driving vehicles. Currently, wire-controlled braking and steering technologies and products have not fully met safety application requirements.
Driver Monitoring Systems (DMS) are primarily used for driver identification, fatigue driving detection, and hazardous behavior monitoring. For L3-level autonomous vehicles, drivers are still required to take over the control of the vehicle in special circumstances, and some national regulations have made specific provisions regarding whether drivers can make phone calls or watch entertainment systems under L3 assisted driving conditions. These requirements necessitate the vehicle to be equipped with DMS to determine responsibility in the event of an accident. Current DMS solutions struggle to meet the demands of L3-level assisted driving.
The industry chain needs adjustment.
As higher-level assisted driving technologies mature, they will have a significant impact on the related chip component industry chain, and may even lead to a reorganization of the industry chain.
Under the traditional distributed E/E architecture, the vehicle's assisted driving system is composed of several independent subsystems (forward ADAS, side and rear ADAS, parking assistance system, panoramic surround view system), each with an ECU, and the main physical structure of the ECU is a microcontroller plus peripheral circuits. In this architecture, hardware and software are tightly coupled, with Tier1 suppliers providing "black box" delivery of integrated hardware and software to the vehicle manufacturers. As the vehicle E/E architecture transitions from distributed to centralized, the ECUs corresponding to the assisted driving subsystems also merge into an assisted driving domain controller, the main control chip evolves from an MCU to a higher-performance SoC heterogeneous chip, and the software architecture upgrades to SOA, which includes three parts: system software (virtual machine, system kernel, middleware), algorithm modules, and the application layer, achieving hardware-software decoupling. Consequently, the entire assisted driving system industry chain is also broken down into several major segments: chips, hardware integration and production, software development, algorithm development, and applications.
In the early stages of industry transformation, segments such as chips, middleware, and algorithm development have each spawned a number of startups. At this time, the core barrier for related companies lies in whether they have sufficient development capabilities and mass production experience in their respective segments. For example, in recent years, Desay SV Automotive has secured numerous orders from car manufacturers based on its mass production experience with an assisted driving domain controller based on the NVIDIA Orin chip. However, as some mid-low computing power platform domain controllers gradually become standardized, the requirements for excellent Tier1 enterprises (including chip suppliers, integration suppliers, algorithm suppliers, etc.) are no longer limited to a single segment of the industry chain. More importantly, they need to leverage their current leading advantages to fully integrate the upstream and downstream of the industry chain, and as much as possible, possess an integrated supply capability that includes chips, algorithms, manufacturing, and more.
Perception algorithms and software technology still need to advance.
Led by Tesla, vehicle manufacturers, especially those in China, have been reconstructing their perception architecture since 2022 and have proposed "heavy perception, light mapping" technical solutions. However, even with the support of a new perception architecture, the intelligent driving system still cannot rely solely on ordinary navigation maps like humans do. Currently, it only reduces the dependence on high-precision maps, rather than completely abandoning the use of any form of maps. The incremental information contained in high-precision maps can be divided into two categories: one is high-precision road geometric data (such as road width, route curvature radius, etc.), and some intelligent driving manufacturers can already supplement this information through BEV real-time perception; the other is road topology structure (such as the connection relationships between lanes, the binding of intersection traffic lights and corresponding lanes, etc.), and intelligent driving manufacturers are temporarily unable to supplement the above information solely through the BEV network architecture.
Neural network-based assisted driving models are set up with algorithm frameworks by engineers and rely on a large amount of data for parameter updates and tuning. Therefore, the level of a vehicle manufacturer's intelligent driving = algorithm construction capability * data training efficiency, where the algorithm construction capability is determined by factors such as the choice of the model itself and the technical route, and data training efficiency is determined by the vehicle manufacturer's data closed-loop capability. Currently, there are still significant differences in the maturity and iteration efficiency of data closed-loop construction among various vehicle manufacturers, so the data closed-loop capability will directly determine the vehicle manufacturer's level of intelligent driving.
The efficiency of a vehicle manufacturer's data closed-loop will be determined by its engineering capabilities. The entire data closed-loop system includes data acquisition, data preprocessing, annotation, simulation, model deployment, etc., and there are many engineering issues that need to be optimized in each link.Refine Regulations and Unify Standards
The current state of autonomous driving technology is at Level 2 (L2) and is progressing towards Level 3 (L3). Unlike L2, L3 is no longer considered an assistive driving feature but a conditional autonomous driving capability. Drivers are not required to constantly monitor the surrounding road conditions and be prepared to take over at any moment. In this case, the responsibility for driving the vehicle shifts more to the vehicle itself. Therefore, traffic accidents that occur when the autonomous driving system is functioning normally should be the responsibility of the vehicle manufacturer. However, looking at the current traffic policies of various countries, L3 autonomous driving technology has not been widely recognized, and the primary responsibility for traffic accidents is mostly clearly identified as the driver. It is evident that governments around the world are cautious about the implementation of autonomous driving, and the pace of enacting relevant laws and regulations is relatively slow, which to some extent restricts the development of higher-level autonomous driving technology.
The implementation of higher-level autonomous driving also requires countries to formulate relevant policies to provide clear answers regarding the identification standards, technical specifications, professional terminology, and evaluation systems for autonomous driving, and to establish a standard system. The establishment of a standard system is very complicated and requires continuous optimization, which poses a significant challenge for governments worldwide.
02
Conclusion
ADAS technology has been developed for many years, and there are increasingly more vehicles on the road equipped with this feature. However, safety issues have not been resolved. Moreover, some vehicles and ADAS systems are, to some extent, "experimenting" on the highway, which carries a considerable risk.
As the level of assistive driving increases, the importance of safety issues becomes more prominent. To elevate the ADAS system to a higher level of application, various hardware and software issues, intelligent perception issues, as well as the formulation of regulations and industry standards, must be thoroughly resolved.
Of course, to achieve Level 4 (L4) or even Level 5 (L5) driving functions in future consumer vehicles, in addition to technological breakthroughs, cost is an inescapable challenge. This is because to achieve L5-level driving, a wide variety of expensive hardware must be equipped on the vehicle, and the software services will not be cheap either. Whether such costs can be popularized in the lives of the general consumer is a question.